Behavior Learning
Research Team
Research Summary
Recently, devices like smart speakers, which understand human language, have become more common. But when people interact, they use more than just words – they also rely on gestures, eye contact, and other nonverbal cues. Our team is researching how these different forms of “interaction” work together so that we can build technology that feels more natural and engaging.
For example, smooth conversations depend on timing and rhythm. We’re developing methods to analyze these “timing shifts” in dialogue to better understand what makes a conversation feel natural and connected. Using what we learn, we’re also creating models that can teach a computer-operated avatar to communicate in more lifelike ways.
Some specific areas we’re working on include:
- Interaction Feature Analysis: Understanding what makes interactions smooth and natural.
- Conversation Generation Models: Developing technology to generate movements for CG avatars so they can engage in realistic conversations.
- Main Research Fields
-
- Human robot interaction
- Machine learning
- Keywords
-
- Reinforcement learning
- Human robot interaction
- Communicative robot
- Motion generation
- Intrinsic motivation
- Research theme
-
- Reinforcement learning of robots interacting with humans.
- Automatic generation of human-like and natural motion of communicative robot based on human cognition.
- Interactive robot operation mechanism through communication.
- Autonomous robots operating in everyday environments.
Yutaka Nakamura
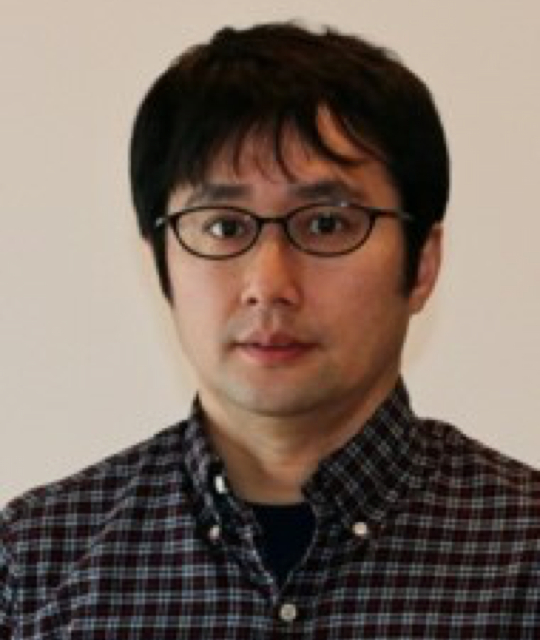
History
- 2004
- Nara institute of science and technology
- 2006
- Osaka University
- 2020
- RIKEN
Members
- Huthaifa Ahmad
- Research Scientist
- Liliana Villamar Gomez
- Special technical staff
- Yuya Okadome
- Visiting Scientist
- Chenfei Xu
- Junior Research Associate
- Siyu Wang
- Research Part-time Worker I
- Zhichao Chen
- Student Trainee
- Zicheng Zhao
- Student Trainee
- Xuhai Li
- Student Trainee
- Takahiro Shibasaki
- Student Trainee
- Yuto Hattori
- Student Trainee
Former member
- Yusuke Nishimura
- Research Part-time Worker I and Student Trainee(2021/01-2023/03)
- Yazan Alkatshah
- Research Part-time Worker I and Student Trainee(2023/05-2025/3)
- Shota Takashiro
- Administrative Part-time Worker II (2021/12-2022/03)
- Duong Nam Tran
- Student Trainee(2024/8-2024/09)
- Haoyang Jiang
- Student Trainee(2024/06-2025/3)
- Bang Trong Nguyen
- Student Trainee(2024/07-2025/3)
- Nayuta Arai
- Research Intern(2023/09)
- Sara Pia Calvitto
- Research Intern(2023/08-2023/11)
Research results
Learning from human demonstration
For real-world human-robot interaction (HRI), it is difficult to hand-craft all the rules for robots owing to diverse situations. Under the circumstances, deep learning approaches should be considered to assist robots in mastering HRI skills. Here, we demonstrate a practical HRI application, in which an android robot acts as a mall receptionist to encourage customers to perform hand hygiene using a hand sanitizer. By learning from the human demonstration, the IRL-driven android achieves a competitive performance to a well-trained human operator. In addition, we construct a IRL-to-RL transition framework to further enhance the android, of which the performance eventually outcompetes the human expert’s with extra data sampling.
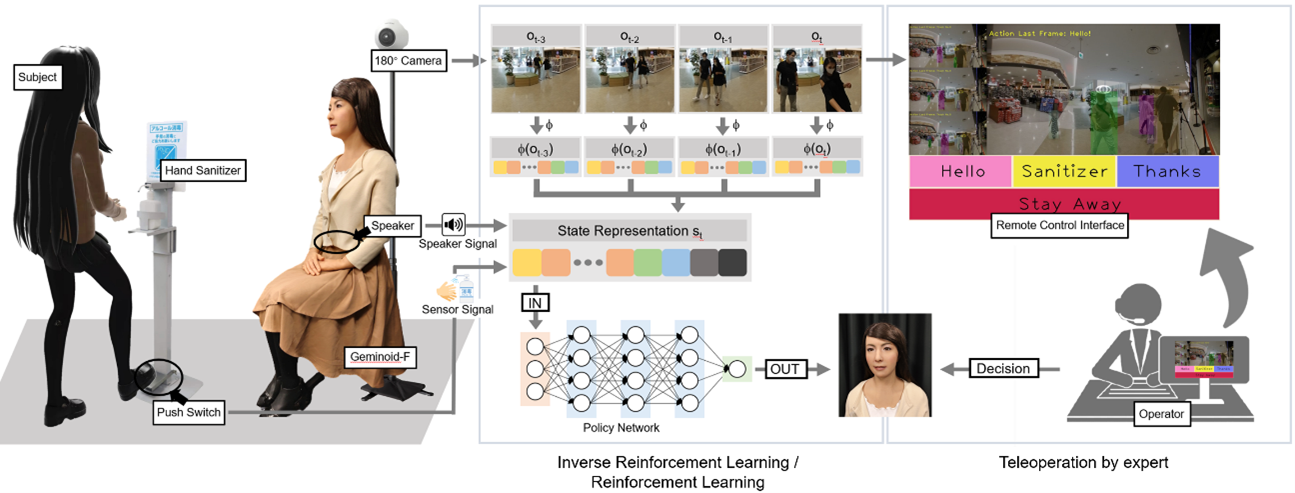
Selected Publications
-
Yuya Okadome and Yutaka Nakamura,
"Feature extraction method using lag operation for sub-grouped multidimensional time series data."
IEEE Access, Volume: 12, Page(s): 98945 – 98959(2024) -
Huthaifa Ahmad, Yutaka Nakamura
”A Study on Designing a Robot with Body Features Tailored for Coexistence with Humans in Daily Life Environments."
33rd IEEE International Conference on Robot and Human Interactive Communication (ROMAN)(2024) -
Zhichao Chen, Yutaka Nakamura, Hiroshi Ishiguro,
“Outperformance of Mall-Receptionist Android as Inverse Reinforcement Learning Is Transitioned to Reinforcement Learning,”
IEEE Robotics and Automation Letters. ( open access ) -
岡留 有哉, 阿多 健史郎, 石黒 浩, 中村 泰
”対話中の振る舞い予測のための時間的整合性に注目した自己教師あり学習”
人工知能学会論文誌 (2022) -
Zhichao Chen, Nakamura Yutaka, Hiroshi Ishiguro
"Android As a Receptionist in a Shopping Mall Using Inverse Reinforcement Learning"
IEEE/RSJ International Conference on Intelligent Robots and Systems (2022) -
Naoki Ise, Yoshihiro Nakata, Yutaka Nakamura, Hiroshi Ishiguro
"Gaze motion and subjective workload assessment while performing a task walking hand in hand with a mobile robot"
International Journal of Social Robotics (2022) -
Huthaifa Ahmad, Yutaka Nakamura
"A robot that is always ready for safe physical interactions"
Interdisciplinary Conference on Mechanics, Computers and Electrics (ICMECE 2022) -
Satoshi Yagi, Yoshihiro Nakata, Yutaka Nakamura, Hiroshi Ishiguro
"Can an android's posture and movement discriminate against the ambiguous emotion perceived from its facial expressions?"
Plos ONE (2021) -
Yusuke Nishimura, Yutaka Nakamura, and Hiroshi Ishiguro
"Human interaction behavior modeling using Generative Adversarial Networks"
Neural Networks, 132, pp.521—531 (2020) -
Ahmed Hussain Qureshi, Yutaka Nakamura, Yuichiro Yoshikawa, and Hiroshi Ishiguro
"Intrinsically motivated reinforcement learning for human-robot interaction in the real-world"
Neural Networks, 107, pp. 23—33 (2018) -
Yuya Okadome, Yutaka Nakamura, and Hiroshi Ishiguro
"A confidence-based roadmap using Gaussian process regressio"
Autonomous Robots, 41(4) (2017) -
Yutaka Nakamura, Takeshi Mori, Masa-aki Sato, and Shin Ishii
"Reinforcement learning for a biped robot based on a CPG-actor-critic method"
Neural Networks, 20(6), pp.723-735 (2007)
Links
Behavior Learning Research Team(RIKEN)
Contact Information
yutaka.nakamura [at] riken.jp